Back in 2008 my ex-co-blogger Eliezer Yudkowsky and I discussed his “AI foom” concept, a discussion that we recently spun off into a book. I’ve heard for a while that Nick Bostrom was working on a book elaborating related ideas, and this week his Superintelligence was finally available to me to read, via Kindle. I’ve read it now, along with a few dozen reviews I’ve found online. Alas, only the two reviews on GoodReads even mention the big problem I have with one of his main premises, the same problem I’ve had with Yudkowsky’s views. Bostrom hardly mentions the issue in his 300 pages (he’s focused on control issues).
All of which makes it look like I’m the one with the problem; everyone else gets it. Even so, I’m gonna try to explain my problem again, in the hope that someone can explain where I’m going wrong. Here goes.
“Intelligence” just means an ability to do mental/calculation tasks, averaged over many tasks. I’ve always found it plausible that machines will continue to do more kinds of mental tasks better, and eventually be better at pretty much all of them. But what I’ve found it hard to accept is a “local explosion.” This is where a single machine, built by a single project using only a tiny fraction of world resources, goes in a short time (e.g., weeks) from being so weak that it is usually beat by a single human with the usual tools, to so powerful that it easily takes over the entire world. Yes, smarter machines may greatly increase overall economic growth rates, and yes such growth may be uneven. But this degree of unevenness seems implausibly extreme. Let me explain.
If we count by economic value, humans now do most of the mental tasks worth doing. Evolution has given us a brain chock-full of useful well-honed modules. And the fact that most mental tasks require the use of many modules is enough to explain why some of us are smarter than others. (There’d be a common “g” factor in task performance even with independent module variation.) Our modules aren’t that different from those of other primates, but because ours are different enough to allow lots of cultural transmission of innovation, we’ve out-competed other primates handily.
We’ve had computers for over seventy years, and have slowly build up libraries of software modules for them. Like brains, computers do mental tasks by combining modules. An important mental task is software innovation: improving these modules, adding new ones, and finding new ways to combine them. Ideas for new modules are sometimes inspired by the modules we see in our brains. When an innovation team finds an improvement, they usually sell access to it, which gives them resources for new projects, and lets others take advantage of their innovation.
Since software is often fragile and context dependent, much innovation consists of making new modules that are rather similar to old ones, except that they work in somewhat different contexts. We try to avoid this fragility via abstraction, but this is usually hard. Today humans also produce most of the value in innovations tasks, though software sometimes helps. We even try to innovate new ways to innovate, but that is also very hard.
Overall we so far just aren’t very good at writing software to compete with the rich well-honed modules in human brains. And we are bad at making software to make more software. But computer hardware gets cheaper, software libraries grow, and we learn more tricks for making better software. Over time, software will get better. And in centuries, it may rival human abilities.
In this context, Bostrom imagines that a single “machine intelligence project” builds a “system” or “machine” that follows the following trajectory:
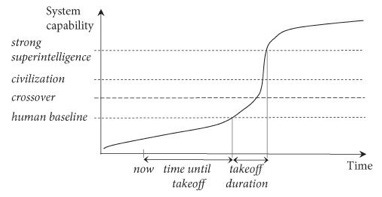
“Human baseline” represents the effective intellectual capabilities of a representative human adult with access to the information sources and technological aids currently available in developed countries. … “The crossover”, a point beyond which the system’s further improvement is mainly driven by the system’s own actions rather than by work performed upon it by others. … Parity with the combined intellectual capability of all of humanity (again anchored to the present) … [is] “civilization baseline”.
These usual “technological aids” include all of the other software available for sale in the world. So apparently the reason the “baseline” and “civilization” marks are flat is that this project is not sharing its innovations with the rest of the world, and available tools aren’t improving much during the period shown. Bostrom distinguishes takeoff durations that are fast (minutes, hours, or days), moderate (months or years), or slow (decades or centuries) and says “a fast or medium takeoff looks more likely.” As it now takes the world economy fifteen years to double, Bostrom sees one project becoming a “singleton” that rules all:
The nature of the intelligence explosion does encourage a winner-take-all dynamic. In this case, if there is no extensive collaboration before the takeoff, a singleton is likely to emerge – a single project would undergo the transition alone, at some point obtaining a decisive strategic advantage.
Bostrom accepts there there might be more than one such project, but suggests that likely only the first one would matter, because the time delays between projects would be like the years and decades we’ve seen between when different nations could build various kinds of nuclear weapons or rockets. Presumably these examples set the rough expectations we should have in mind for the complexity, budget, and secrecy of the machine intelligence projects Bostrom has in mind.
In Bostrom’s graph above the line for an initially small project and system has a much higher slope, which means that it becomes in a short time vastly better at software innovation. Better than the entire rest of the world put together. And my key question is: how could it plausibly do that? Since the rest of the world is already trying the best it can to usefully innovate, and to abstract to promote such innovation, what exactly gives one small project such a huge advantage to let it innovate so much faster?
After all, if a project can’t innovate faster than the world, it can’t grow faster to take over the world. Yes there may be feedback effects, where better software makes it easier to make more software, speeds up hardware gains to encourage better software, etc. But if these feedback effects apply nearly as strongly to software inside and outside the project, it won’t give much advantage to the project relative to the world. Yes by isolating itself the project may prevent others from building on its gains. But this also keeps the project from gaining revenue to help it to grow.
A system that can perform well across a wide range of tasks probably needs thousands of good modules. Same for a system that innovates well across that scope. And so a system that is a much better innovator across such a wide scope needs much better versions of that many modules. But this seems like far more innovation than is possible to produce within projects of the size that made nukes or rockets.
In fact, most software innovation seems to be driven by hardware advances, instead of innovator creativity. Apparently, good ideas are available but must usually wait until hardware is cheap enough to support them.
Yes, sometimes architectural choices have wider impacts. But I was an artificial intelligence researcher for nine years, ending twenty years ago, and I never saw an architecture choice make a huge difference, relative to other reasonable architecture choices. For most big systems, overall architecture matters a lot less than getting lots of detail right. Researchers have long wandered the space of architectures, mostly rediscovering variations on what others found before.
Some hope that a small project could be much better at innovation because it specializes in that topic, and much better understands new theoretical insights into the basic nature of innovation or intelligence. But I don’t think those are actually topics where one can usefully specialize much, or where we’ll find much useful new theory. To be much better at learning, the project would instead have to be much better at hundreds of specific kinds of learning. Which is very hard to do in a small project.
What does Bostrom say? Alas, not much. He distinguishes several advantages of digital over human minds, but all software shares those advantages. Bostrom also distinguishes five paths: better software, brain emulation (i.e., ems), biological enhancement of humans, brain-computer interfaces, and better human organizations. He doesn’t think interfaces would work, and sees organizations and better biology as only playing supporting roles.
That leaves software and ems. Between the two Bostrom thinks it “fairly likely” software will be first, and he thinks that even if an em transition doesn’t create a singleton, a later software-based explosion will. I can at least see a plausible sudden gain story for ems, as almost-working ems aren’t very useful. But in this post I’ll focus on software explosions.
Imagine in the year 1000 you didn’t understand “industry,” but knew it was coming, would be powerful, and involved iron and coal. You might then have pictured a blacksmith inventing and then forging himself an industry, and standing in a city square waiving it about, commanding all to bow down before his terrible weapon. Today you can see this is silly — industry sits in thousands of places, must be wielded by thousands of people, and needed thousands of inventions to make it work.
Similarly, while you might imagine someday standing in awe in front of a super intelligence that embodies all the power of a new age, superintelligence just isn’t the sort of thing that one project could invent. As “intelligence” is just the name we give to being better at many mental tasks by using many good mental modules, there’s no one place to improve it. So I can’t see a plausible way one project could increase its intelligence vastly faster than could the rest of the world.
(One might perhaps move a lot of intelligence at once from humans to machines, instead of creating it. But that is the em scenario, which I’ve set aside here.)
So, bottom line, much of Nick Bostrom’s book Superintelligence is based on the premise that a single software project, which starts out with a tiny fraction of world resources, could within a few weeks grow so strong to take over the world. But this seems to require that this project be vastly better than the rest of the world at improving software. I don’t see how it could plausibly do that. What I am I missing?
Added 2Sep: See also related posts after: Irreducible Detail, Regulating Infinity.
Added 5Nov: Let me be clear: Bostrom’s book has much thoughtful analysis of AI foom consequences and policy responses. But aside from mentioning a few factors that might increase or decrease foom chances, Bostrom simply doesn’t given an argument that we should expect foom. Instead, Bostrom just assumes that the reader thinks foom likely enough to be worth his detailed analysis.
Do you know of anyone doing explicit economic models of FOOM like scenarios? I'm starting to think about them here https://modellingselfmodification.wordpress.com/2024/04/07/notes-on-an-approach-to-modelling-ai-self-modification/
As I re-review the intelligence explosion / singularity discussions, I find myself thinking * Intelligence explosion depends on what you define as intelligence * ... I juxtapose Lex Friedman's points "most of the big questions of intelligence have not been answered nor properly formulated" [see Lex Friedman, 2nd slide of Deep Learning Basics Slide Deck, Spring 2019, deeplearning.mit.edu] ...
So if you want to declare look at Moore's law, all you can really talk about is computing power, not intelligence. I am surveying the assorted definitions of intelligence ... and guess what ... we've got a long ways to go.
More later ..